Predicting the Recovery of Psychophysiological State after Chronic Stress: A Neural Network Model
V.I. Tsymbalyuk1, S.N. Vadzyuk2, P.S. Tabas2, O.M. Ratynska2, V.V. Sas2, M.O. Sopiga3
- Institute of Neurosurgery of the National Academy of Medical Sciences of Ukraine, Kyiv, Ukraine;
- Ternopil National Medical University named after I.Ya. Horbachevsky, Ukraine;
- National University of Life Resources and Environmental Sciences of Ukraine; Kyiv, Ukraine
DOI: https://doi.org/10.15407/fz71.02.003
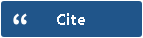
Abstract
The psychophysiological state of combatants is determined by
stress resistance, volitional self-regulation, the level of per-
sonal anxiety and individual characteristics of higher nervous
activity. Scientists have so far paid insufficient attention to
predicting the recovery of the psycho-emotional state, although
it is extremely important in modern conditions. The purpose of
the research is to study the features of the psychophysiological
state of individuals with different stress resistance who have
experienced chronic stress and to develop neural network
models for predicting the effectiveness and duration of their re -
habilitation. An examination of 637 people who returned from
the combat zone in the first week, one month and three months
later was conducted. Stress resistance, level of depression,
volitional self-regulation, personal and situational anxiety were
determined, and individual characteristics of higher nervous
activity were assessed. During the study, it was established
that the most significant psychophysiological parameters for
predicting an effective rehabilitation period are stress resis-
tance, personal and situational anxiety, and emotional stability.
These parameters had a pronounced impact on forecasting with
high accuracy (92%), sensitivity (90%). The high sensitivity
and specificity of the constructed neural model allows for an
individual approach to the duration of the rehabilitation period
for persons who have experienced acute stress.
Keywords:
stress resistance; chronic stress; psychophysiological state; neural networks; forecasting; rehabilitation
References
- Turinina OL. Psychology of traumatic situations: training manual for students in higher education. Kyiv: Person; 2017. [Ukrainian].
- Tkachuk IM. Prevalence of anxiety and depressive disorders in servicemen participating in anti-terrorist operations. Herald Sci Res. 2018; 1:64-6.
- Berezovska L, Bohaichuk V . Emotional stability of mili-tary officers: an empirical perspective. Bull Natl Defense Univ. 2022; 9:19-25.
- Bartone PT, Homish GG. Influence of hardiness, avoidance coping, and combat exposure on depression in returning war veterans: A moderated-mediation study. J Affect Disord. 2020; 265:511-8.
CrossRef
PubMed
- Karstoft KI, Nielsen ABS, Nielsen T. Assessment of depression in veterans across missions: a validity study using Rasch measurement models. EJPT. 2017; 8(1):65-8.
CrossRef
PubMed PubMedCentral
- Moradi Y , Dowran B, Sepandi M. The global prevalence of depression, suicide ideation, and attempts in the military forces: a systematic review and meta-analysis of cross sectional studies. BMC Psychiatr. 2021; 21(1):510.
CrossRef
PubMed PubMedCentral
- Vadzyuk SN, Sas VV . The state of depression and anxiety in military personnel with different stress resistance. Arch Clin Exp Med. 2023; 3:46-55.
- V ogelzangs N, De Jonge P, Smit JH, Bahn S, Penninx BW. Cytokine production capacity in depression and anxiety. Transl Psychiatr. 2016; 6(5):825-e825.
CrossRef
PubMed PubMedCentral
- Smith BW, Dalen J, Wiggins K, Tooley E, Christopher P, Bernard J. The brief resilience scale: Assessing the ability to bounce back. Int J Behav Med. 2008:194-200.
CrossRef
PubMed
- Kocalevent RD, Hinz A, Brähler E. Standardization of the depression screener patient health questionnaire (PHQ- 9) in the general population. Gen Hosp Psychiatr. 2013; 35(5):551-5.
CrossRef
PubMed
- Ustinov OV . Algorithm of a doctor's actions when pro-viding medical care to patients with depression. UMJ. 2015; 2:1-12.
- Alonso-Tapia J, Calderón EP, Ruiz MA. Development and validity of the emotion and motivation self-regulation questionnaire (EMSR-Q). Span J Psychol. 2014; 17:55.
CrossRef
PubMed
- Rab SL, Admon R. Parsing inter-and intra-individual variability in key nervous system mechanisms of stress responsivity and across functional domains. Neurosci Biobehav Rev. 2021; 120:550-64.
CrossRef
PubMed
- Spielberger CD, Gonzalez-Reigosa F, Martinez-Urrutia A, Natalicio LF, Natalicio DS. The state-trait anxiety inventory. IJP. 1971; 5.
- Kelleher JD. Deep learning. Massachusetts (USA): MIT press; 2019.
CrossRef
- Palatnik de Sousa I, Maria Bernardes Rebuzzi Vellasco M, Costa da Silva E. Local interpretable model-agnostic explanations for classification of lymph node metastases. Sensors. 2019; 19(13):2969.
CrossRef
PubMed PubMedCentral
- Giorgi FM, Ceraolo C, Mercatelli D. The R language: an engine for bioinformatics and data science. Life. 2022; 12(5):648.
CrossRef
PubMed PubMedCentral
- Pang B, Nijkamp E, Wu YN. Deep learning with tensor flow: A review. JEBS. 2020; 45(2):227-48.
CrossRef
|