|
|
|
|
PREDICTION OF BLOOD PRESSURE LEVEL USING NEURAL NETWORK MODELS
S.N. Vadzyuk, P.S.Tabas
I. Horbachevsky Ternopil National Medical University, Ukraine
DOI: https://doi.org/10.15407/fz70.06.017
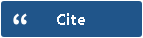
Abstract
Psychophysiological changes are common factors contributing
to the development of arterial hypertension. The aim of this
study was to examine the psychophysiological characteristics
of individuals with different levels of blood pressure (BP), to
build and compare the predictive accuracy of a classical neural
network and a Kolmogorov-Arnold network in forecasting
BP levels, as well as to determine the prognostic value of
the studied psychophysiological parameters. The study
involved 240 practically healthy individuals aged 18 to 22.
All participants underwent initial BP measurements, based
on which they were divided into three groups according to
the recommendations of the European Society of Cardiology.
The research included 24-h BP monitoring, an assessment
of anxiety levels, and evaluations of well-being, activity,
mood, and sleep quality. Predictive models were created
from the obtained data to forecast systolic BP levels >130
mmHg. Situational and trait anxiety levels were found to
be significantly higher in individuals with normal-high BP
compared to those with normal-low and normal BP levels.
Sleep quality, measured by the PSQI (Pittsburgh sleep quality
index) questionnaire, was significantly lower in individuals
with normal-high BP compared to the other groups. The
neural network constructed in this study demonstrated that
psychophysiological indicators can be effectively used for
predicting elevated BP levels and for the early diagnosis of
arterial hypertension. This research is the first to apply the
Kolmogorov-Arnold network for predicting high BP levels.
The study found that this network was highly effective,
outperforming the multilayer perceptron with a larger number
of neurons in terms of predictive accuracy.
Keywords:
blood pressure prediction; anxiety; sleep quality; neural network; Kolmogorov-Arnold network.
References
- Tsao CW, Aday AW, Almarzooq ZI, Alonso A, Beaton AZ, Bittencourt MS, et al. Heart disease and stroke statistics - 2022 update: A report from the American Heart Association. Circulation. 2022;145(8).
- Fuchs FD, Whelton PK. High blood pressure and cardiovascular disease. Hypertension. 2020;75(2):285-92.
CrossRef
PubMed PubMedCentral
- Vaughan AS, Coronado F, Casper M, Loustalot F, Wright JS. County-Level Trends in Hypertension-Related Cardiovascular Disease Mortality-United States, 2000 to 2019. JAHA. 2022;11(7).
CrossRef
PubMed PubMedCentral
- Zhang H, Li Y, Zhao X, Mao Z, Abdulai T, Liu X, et al. The association between PSQI score and hypertension in a Chinese rural population: The henan rural cohort study. Sleep Med. 2019;58:27-34.
CrossRef
PubMed
- Kolahi P, Salehi M, Madahi ME, Sepahmansour M. Effectiveness of mindfulness-based stress reduction on state/trait anxiety and hypertension in patients with coronary heart disease with hypertension. Adv Cogn Psychol. 2022;24(1).
- Cash RE, Anderson SE, Lancaster KE, Lu B, Rivard MK, Camargo CA, et al. Associations between sleep, stress, and cardiovascular health in emergency medical services personnel. JACEP Open. 2021;2(4).
CrossRef
PubMed PubMedCentral
- Lim LF, Solmi M, Cortese S. Association between anxiety and hypertension in adults: A systematic review and metaanalysis. Neurosci Biobehav Rev. 2021;131:96-119.
CrossRef
PubMed
- Wallace ML, Coleman TS, Mentch LK, Buysse DJ, Graves JL, Hagen EW, et al. Physiological sleep measures predict time to 15 year mortality in community adults: Application of a novel machine learning framework. J Sleep Res. 2021;30(6).
CrossRef
PubMed PubMedCentral
- Ramzy D. Definition of hypertension and pressure goals during treatment (ESC-ESH Guidelines 2018). Eur Soc Cardiol J. 2019;17.
- Niiranen TJ, Henglin M, Claggett B, Muggeo VMR, McCabe E, Jain M, et al. Trajectories of Blood pressure elevation preceding hypertension onset: An analysis of the framingham heart study original cohort. JAMA Cardiol. 2018;3(5):427-31.
CrossRef
PubMed PubMedCentral
- Chowdhury MZI, Naeem I, Quan H, Leung AA, Sikdar KC, O'Beirne M, et al. Prediction of hypertension using traditional regression and machine learning models: A systematic review and meta-analysis. PLoS ONE. 2022;17(4).
CrossRef
PubMed PubMedCentral
- Liu Z, Wang Y, Vaidya S, Ruehle F, Halverson J, Soljačić M, et al. KAN: Kolmogorov-Arnold Networks. arXiv; 2024.
- Abueidda DW, Pantidis P, Mobash er ME. DeepOKAN: Deep operator network based on Kolmogorov Arnold networks for mechanics problems. arXiv; 2024.
- Selitskiy S. Kolmogorov's Gate Non-linearity as a step toward much smaller artificial neural networks: In: Proceedings of the 24th International Conference on Enterprise Information Systems. SCITEPRESS; 2022. p. 492-9.
CrossRef
- Agaev N, Kokun O, Pishko I, Lozinska N, Ostapchuk V, Tkachenko V. Collection of techniques for diagnosis of negative mental states of military personnel. Kyiv, Ukraine: NDC of the State Department of the ZSU; 2016.
- Sheremet IV, Bilyk VG, Vasylenko KS. The influence of the educational process on psycho-emotional state of students as a psychological and pedagogical problem. Ped Educat: Theory Pract. 2020;(28):434-42.
CrossRef
- Liu D, Kahathuduwa C, Vazsonyi AT. The pittsburgh sleep quality index (PSQI): Psychometric and clinical risk score applications among college students. Psychol Assess. 2021;33(9):816-26.
CrossRef
PubMed
- Nohara Y, Matsumoto K, Soejima H, Nakashima N. Explanation of machine learning models using shapley additive explanation and application for real data in hospital. Comp Method Prog Biomed Update. 2022;214:106584.
CrossRef
PubMed
- Anyfanti P, Malliora A, Chionidou A, Mastrogiannis K, Lazaridis A, Gkaliagkousi E. Clinical significance of nocturnal hypertension and nighttime blood pressure dipping in hypertension. Curr Hyperten Rep. 2024;26(2):69-80.
CrossRef
PubMed
- Gavriilaki M, Anyfanti P, Nikolaidou B, Lazaridis A, Gavriilaki E, Douma S, et al. Nighttime dipping status and risk of cardiovascular events in patients with untreated hypertension: A systematic review and meta-analysis. J Clin Hyperten. 2020;22(11):1951-9.
CrossRef
PubMed PubMedCentral
- Yuan Y, Heizhati M, Wang L, Li M, Lin M, Gan L, et al. Poor sleep quality is associated with new-onset hypertension in a diverse young and middle-aged population. Sleep Med. 2021;88:189-96.
CrossRef
PubMed
- Thomas SJ, Booth JN, Jaeger BC, Hubbard D, Sakhuja S, Abdalla M, et al. Association of sleep characteristics with nocturnal hypertension and nondipping blood pressure in the CARDIA study. JAHA. 2020;9(7).
CrossRef
PubMed PubMedCentral
- Nimmala S, Usha Rani R, Nutipalli P, Naresh U, Reddy RR. An intelli BPS: An Intelligent biopsychosocial parametersbased machine learning model to predict hypertension. In: Gunjan VK, Zurada JM, editors. Proceedings of 4th International Conference on recent trends in machine learning, IoT, Smart Cities and Applications. Singapore: Springer Nature Singapore. 2024. 651-61.
CrossRef
|
|
|
|
|
|
|